2025-2026 USC Capital One Center Call for Proposals
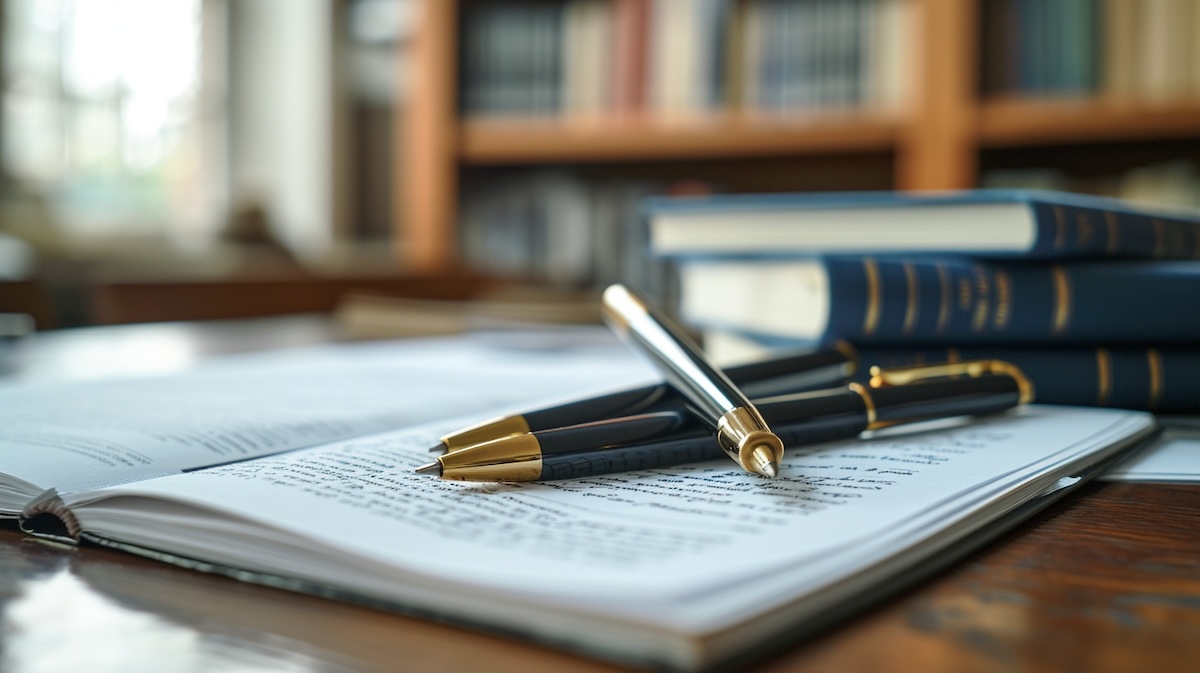
Each year, through a competitive process, the Center will provide support for several research projects focused on the development of new methodologies for AI with potential applications to solving complex financial problems. The Center will also provide annual fellowships to doctoral students working in this research area, enabling them to advance research frontiers. Fellowship recipients will be named as Capital One Fellows in recognition of their promise and achievements. In addition to funded research projects and annual fellowships for doctoral students, the collaborators will host an annual joint public research symposium to share their knowledge with the machine learning and AI communities.
We hereby invite USC faculty to nominate qualifying Ph.D. students for year 2025 Capital One Fellows
Some of the research topics of interest to CREDIF are listed below. It is expected that PhD Fellowships will involve PhD students whose research thesis has some relevance to the topics below:
- Robust AI in the face of noisy data and labels: To train AI/ML models in the presence of noisy and imperfect data, we are interested in the development of a Robust AI framework with focus on enhancing resiliency and accuracy of the AI system that can be built with data anomalies, inconsistencies, collection bias, and under representation of multiple data groups. The robust AI framework should include advanced methodologies for data cleaning, noise reduction, bias detection, and dealing with data drift and data imbalance.
- Proposal should outline integrating these capabilities into AI model training and development workflow. The evaluation criteria should focus on ability to handle imperfect data and model stability.
- Synthetic data generation for training and evaluating GenAI models on difficult tasks, such as reasoning: How might we leverage GenAI or other techniques to generate synthetic data that closely mirrors the characteristics of real-world data? This could be for enhancing training datasets or creating proxy datasets that maintain essential properties while ensuring privacy, allowing the research community to engage in our problems of interest. We’re particularly interested in datasets or techniques that focus on reasoning tasks, such as commonsense reasoning, mathematical reasoning, financial reasoning, complex instruction following, and related areas.
- Explainability framework that improves transparency and interoperability in LLM based systems (particularly in the presence of multiple agents):
- The proposal should highlight unique challenges in explainability in multi-agentic workflow and possible methodologies to enable users to understand the decision-making process inside a complex agentic environment with humans in the loop.
- Integration of graph knowledge representations with AI: Providing accurate and relevant information is key to the success of Generative AI systems. However, standard methods often fall short when dealing with complex questions that require information from various sources. With potential application to financial services, we're interested in methods that leverage graph-based knowledge representation to address these limitations and improve the quality of AI-generated responses.
- Benchmarks for evaluating the capabilities of Large Language Models: Create new metrics, benchmarks, and evaluation methods to measure the performance of LLMs on challenging tasks like reasoning, instruction following, summarization, question answering, etc. These benchmarks could either evaluate general intelligence or focus on specialized domains, such as finance, mathematics, or other specific fields.
Criteria
- Quality of research 55%
- PI qualifications 25%
- Relevance to CREDIF 20%
Full proposals (compiled in a single-file PDF format) should be sent via email to the
administrative program manager Ariana Perez via email (arianape@usc.edu), by 5 pm Pacific time
on 03/21/2025.
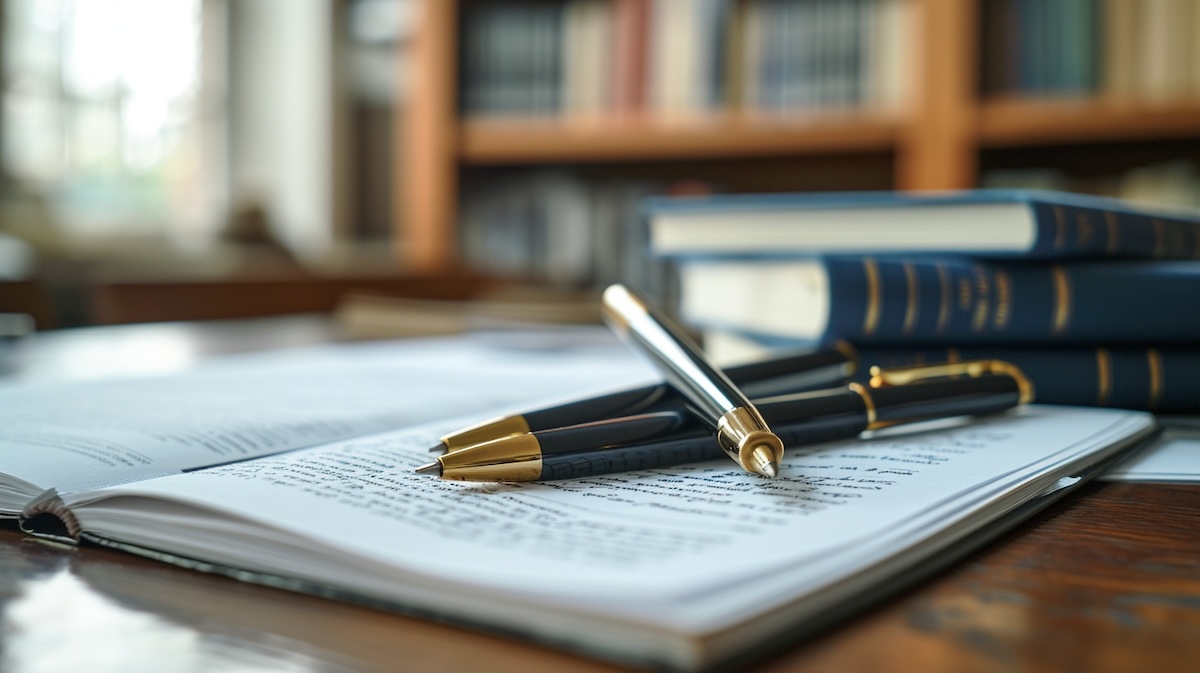